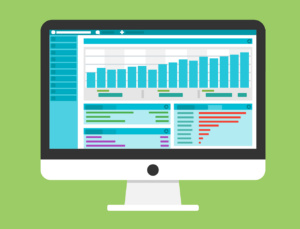
Image by 200 Degrees from Pixabay
What is a HMIS?
A health management information system (HMIS) collects, stores, analyses, and evaluates health-related data from health facility to district, regional and national administrative levels. It provides analytical reports and visualisations that facilitate decision making at all these levels. HMIS are also referred to as routine health information systems.
A HMIS derives much of its information from patient-provider interactions in health facilities. Hospitals, health centres, and community outreach services provide health care across preventive, promotive, medical and surgical, rehabilitation, and palliative care interventions.
The HMIS also collects data from beyond government-run facilities including from non-profit, for-profit, faith-based facilities and from service delivery sites such as prisons, schools, workplaces and communities.
- Facilities collect data – which are integral to the services they provide – to ensure good management of patients.
- Health managers aggregate and report the data to higher administrative levels, for example district, regional and national levels.
- When aggregated, the data provide information for epidemiological surveillance and for monitoring health services performance in terms of access, coverage, quality, and equity at all levels of the health system.
- The information generated show the range and volume of services delivered to the population, including: prevention such as immunisation, antenatal, delivery and postnatal care; treatment of acute conditions such as malaria, diarrhoea, and upper respiratory tract infections; chronic conditions such as HIV, tuberculosis, high blood pressure; and management of surgery and trauma.
- In addition to generating information on interventions – admissions, treatments administered and health outcomes – a HMIS also produces data on the availability of services, infrastructure, equipment and supplies needed to deliver such interventions.
The HMIS provides information for local planning. It also contributes to country level monitoring and evaluation, research, policy and planning and generates indicators about outputs, outcomes and impact.
Why a HMIS?
A HMIS has different and sometimes conflicting functions: operational; informational; and decision-making. The operational and decision-making functions are essential to ensure proper management of health services for patient/clients. The broader utility of the HMIS is to complement data from other HIS sources to build an evidence base for health sector performance assessment and strategic planning.
The main users of HMIS are managers and care providers at district level and below. Executive managers, public policymakers and researchers can also use HMIS data for governance and research.
Main sources of HMIS data
HMISs are complex, reflecting the multifaceted and heterogeneous nature of health care provision and management. They draw on: individual patient records; family record cards; admissions and discharge registers; ward registers and tally sheets; community level records, infrastructure and resource records, records of health interventions delivered in communities, and periodic assessments of health facility infrastructure and resources. We classify these record systems as:
Individual patient record systems
The majority of data that a HMIS collects at health facilities derives from individual records of patient-provider interactions that include for example: patient identification, clinical diagnoses, results of laboratory and diagnostic tests; prescriptions; preventive, promotive, curative and rehabilitative interventions delivered; and payments made.
Managers report summary indicators based on a subset of the data to the next level of the health system which compiles them to produce indicators across facilities.
Most low-and middle-income countries continue to use paper-based systems for individual records. But hospitals increasingly use electronic medical records. Electronic records require advanced technology and networking skills, sophisticated management processes and maintenance that are often not available at remote facilities in low-resource settings.
Facility-based registry systems
Facility-based registers include admission and discharge registers and ward registers. Some registers list and follow particular individuals requiring ongoing management over a period for time, such as antenatal or immunization care registers, or registers of chronic diseases such as cancer. Each register maintains the minimum information necessary to follow-up the patients.
Regular review of registers enables the health team to identify patients who must be actively pursued to assure compliance with treatment interventions, such as completion of immunization, full treatment of tuberculosis, compliance with anti-retroviral regimens, or regular monitoring and control of blood pressure.
Patient registries are useful for monitoring the quality of health services and for capturing treatment interventions. In addition to data that identify individuals, these registries include diagnosis on admission and discharge, results of laboratory tests and treatments. If a patient died in hospital, the registry also provides cause of death assigned according to the standards of the International Classification of Diseases.
Community level record systems
HMISs integrate data from community-based workers who provide health promotion and disease prevention activities. These providers may:
- Work for the health system, for example the Health Extension Workers in Ethiopia or community health workers in Kenya, or
- Work informally as community-based providers, for instance volunteers serving people living with HIV who may or may not be associated with the health system.
The information these providers collect at the point of service is essential for community programme management and decision-making on budget, policies and human resources. Community health workers use data to follow their clients and manage their care, especially for interventions that require longitudinal follow-up and community-facility linkages.
It is important to link community level data to facility-based information systems to avoid double counting of health events. Data collection tools require literacy and numeracy skills. Health facility staff should support and supervise community health workers to ensure properly delegated clinical services, for example to help find clients or patients who are lost to follow-up.
Health facility assessments (HFAs)
Alongside the routine collection of data as a by-product of patient management and facility administration, a HMIS also includes periodic collection of information from health facilities that is not included in routine reports. HFAs generate information on: facility infrastructure, equipment and commodities; human resources; readiness to deliver specific interventions (such as tuberculosis management); and service utilization. HFAs are an efficient way to collect information on facility availability and distribution. They can identify where change is needed to strengthen the health system.
Users and uses of HMIS data
HMIS units at each level of the health system manage data to inform activities at that level and below, and to report a required subset of information to the next highest administrative level. For example, a hospital runs its own information system, which includes management of patients and of the commodities and supplies needed to run the hospital.
Managers at higher administrative levels require health care facilities, through the HMIS, to send regular reports, for example cases of notifiable conditions, numbers of vaccinations administered, antenatal care visits, facility deliveries, and patients seen by diagnosis. The district level manages and coordinates reports from facilities as well as from different programmes. A HIV/AIDS programme, for example, will collate information from facilities on coverage of interventions for prevention of mother-to-child transmission of HIV (PMTCT), and uptake and continuity of antiretroviral treatments.
Routine facility data produce information on outcomes and impact. For indicators such as tuberculosis treatment outcomes, coverage of interventions for PMTCT, and uptake and continuity of antiretroviral treatments, facilities are the sole source of data. The HMIS tracks delivery of clinical treatment for conditions, such as diabetes, hypertension, and cancers, that require long-term follow-up and monitoring of treatment compliance and health outcomes. This is important both from the individual patient perspective, but also for the management of services for these diseases and for programme planning and evaluation. For example, Afghanistan uses a Balanced Scorecard framework to measure the performance of reproductive maternal and child health programs using HMIS data.
While data managers organize collection and management of data, it is often the user who collects the data, for example a physician or nurse who completes the patient records. Managers and users must work closely together to obtain the information users require.
Limitations and challenges
HMIS data in low- and middle-income countries are beset by problems of quality so that end-users do not always trusted them or consider them fit for purpose. Data quality limitations include missing values, measurement errors, and mistakes in data entry and computation. The perception that routine reports from health facilities and districts are often late, incomplete and inaccurate undermines credibility and hampers their use.
Completeness of data
Data from a HMIS are, by definition, limited to those who attend health facilities or use related community-based services. When hard-to-reach groups – such as undocumented migrants, ethnic minorities, the very poor – have difficulty accessing health care, it is important to assess the extent of exclusion and how this will affect the completeness and reliability of HMIS data.
In many low- and middle-income countries, large proportions of the population have severely restricted access to health services because of geographic, economic, and sociocultural barriers. In such settings, facility-based data are not likely to represent the whole population in any given catchment area and the resulting data and indicators will be incomplete and biased.
Where access to care is limited, countries estimate population denominators by extrapolating from the most recent census. Inevitably, such estimates become less reliable over time. This is a particular problem at sub-national levels. It is not unusual to see coverage estimates for indicators such as immunization exceeding 120 per cent for some districts while in others coverage is at unlikely low levels (below 80 per cent). While this may, in part be due to inaccuracies in the numerators (numbers of immunised children) studies have found that it is more often a problem of over-estimation or under-estimation of the target population.
Methods to determine the adequacy of the population data used in evaluating the performance of health indicators, such as coverage involve assessment of the internal validity of the HMIS data, such as completeness of reporting from facilities and districts, as well as comparisons with external sources such as household surveys. For example, in Liberia used Lot Quality Assurance Sampling (LQAS) to compare intervention coverage rates obtained from HMIS data with those obtained through a health outcome survey.
Quality of data sources
HMIS managers need to undertake regular quality assessments of the relative strengths and weaknesses of the data sources. Adjustments of reported data to take into account incomplete reporting and missing values can help increase confidence in and utility of facility-based data. But such adjustments must be based on scientific methods and made transparent to users.
The Data Quality Review (DQR) Toolkit supports a comprehensive review of HMIS data quality, mainly from facilities, and consists of three components:
- A guide for conducting monthly reviews of data quality with immediate checks and feedback so that errors can be identified and corrected as they occur.
- An annual independent assessment of core indicators to identify gaps and errors in reporting and assess the plausibility of reported trend data.
- Periodic in-depth programme-specific reviews timed to feed into programme planning.
The reviews focus on a limited set of tracer indicators covering maternal health, immunization, HIV, tuberculosis and malaria. But countries can include other tracer indicators if needed. Data quality metrics include completeness, timeliness, consistency and accuracy.
Innovation and transformation
For years, health facilities and community health workers have collected data using paper forms or logbooks. This involves laborious and time-consuming data aggregation and compilation, transcription errors, inadequate analysis and visualization, difficulty in data sharing, and poor data storage and retrieval.
To address such challenges, HMISs now use information and communication technology for data collection, aggregation, reporting, storage, and analysis, visualization and dissemination. This revolution has yet to reach all countries and all levels of the health system. But information and communication technology can improve routine, facility-based and administrative data collection, management and use for policy and planning.
Although information and communication technology facilitates HMIS functionality, health managers need to select hardware and software appropriate to their country’s infrastructure, capacity, and resource availability.
Electronic HMIS
Sophisticated and powerful data management applications are available for facilities to use to manage their data. For example,
- The District Health Information System (DHIS2) developed by the University of Oslo. DHIS2 permits data capture on multiple fixed and mobile devices. Because the system allows users to enter data offline, it can be used in locations with poor connectivity. DHIS2 Academies facilitate sharing of experiences. They also strengthen national and regional capacities to successfully set up, design and maintain DHIS2 systems.
- The iHRIS software is an application in support of human resources data management.
- The eLMIS supports logistics and commodities data management. In addition to data entry, data aggregation and storage functions, these applications mostly have a decision support module that can produce routine or ad hoc reports, as well as tailored data visualization products called data dashboards.
Decision support tools
Electronic data management facilitates production of summary analyses and visualisations that are readily understandable by non-technical users, or decision support tools, for example:
- Comparison is a useful analytical method. Comparisons may be: spatial (by health facility; district or province); temporal (trends by week, month or year); indicator-specific (between inputs and outputs); or benchmarked (expected versus achieved results). Comparisons can identify areas or groups that are disadvantaged or failing to achieve expected benchmarks and requiring remedial interventions. Whereas cross-country comparisons of key indicators can be of interest, national decision makers often prefer to limit external comparisons to countries at similar levels of development.
- The four Ts: Trends (progress made), Trajectories (whether the direction of change is positive or negative), Triggers (minimum or maximum acceptable levels at which action needs to be taken) and Targets (indicator levels to be achieved).
- Data dashboards with summary tables, graphs, and other visualizations can illustrate such analyses, showing progress towards goals and identify issues for health programmes to address.
- Geographic Information Systems (GIS) are powerful tools to analyse, organize, and present spatial data in maps.
RHINoVision is an example of an electronic Decision Support Tool. It was developed under the MEASURE Evaluation Project as an electronic dashboard that allows further analysis of HMIS data.
Data architecture to link systems
- A National Health Data Dictionary (NHDD) provides a common language for health policymakers, managers and care providers to communicate and exchange health information in a standard manner. The NHDD develops metadata to harmonise data definitions of commonly used data and indicators. It facilitates mapping of definitions to international standards, such as the International Classification of Diseases or the Systemized Nomenclature for Medicine (SNOMED-CT).
The NHDD requires a sound governance mechanism involving health, statistics and other relevant entities. For example, in Australia, the National Health Information Standards and Statistics Committee oversees development of health metadata standards. A NHDD can be hosted on a software platform, ideally open software solutions such as the Open Concept Lab.
- A data warehouse is a centralized data storage system that facilitates integration of data into one, usually virtual, location, linking the data from all data sources via information exchange protocols. This makes it possible to bring together data across health facilities at different levels, including from patient records and human resource management systems.
Highly developed warehouses incorporate data from sources other than the HMIS, such household surveys or the census. If each individual has a unique identifier, then the system can link data on the same individual across different systems, such as health care, medical insurance and social security.
Developing a data warehouse is a major technological and analytical undertaking. It requires skills of health analysts, statisticians, computer technicians, and data scientists. Once established, a warehouse can benefit patients, providers, health facilities and the entire health system.
Notwithstanding the potential of these digital innovations, in many settings facility-based data collection and transfer are predominantly paper-based. The architecture should be designed to evolve and be relevant across locations and levels of the health system, ready to become more granular and comprehensive with time.
Creating a culture for using HMIS data
Many countries issue annual reports based on HMIS data. But too often decision-makers do not use the information to improve health system performance. Poor use of information is not only due to technical issues, but also results from organizational and behavioural barriers. Hierarchically organized health systems can leave managers at lower levels powerless to use the data. Health professionals, while generally well-prepared for diagnosis and problem identification, are not trained for this type of problem solving. The question is how to build a culture of information use.
The Routine Health Information Network (RHINO) was created in 2001 under the USAID-funded MEASURE Evaluation Project with support by WHO, the World Bank, and John Snow, Inc.. The more than 1,000 RHINO members represent developing country governments, donor agencies, technical groups, and private voluntary organizations. The shared purpose of all these organizations is the effective collection and use of HMIS generated information especially at the district level and below. RHINO as a broader worldwide advocacy and knowledge management organization promotes behavioral change for better use of information in decision making.
MEASURE Evaluation developed the Performance of Routine Information System Management (PRISM) for assessing the reliability and timeliness of routine health information systems in making evidence-based decisions. It has published a set of tools on its website.
The private sector uses human-centered design (HCD) for product and technology development to better understand users’ needs and involve them early in the design of solutions. HCD is a collaborative problem-solving approach that provides broadly applicable methods for developing in-depth understanding of human behaviour. HCD could be applied to establish a culture of using health information, together with other interventions such as: role modeling by senior managers to promote use of data at the district level and below; incentive-based systems to promote use of information such as performance-based financing schemes; allocation of resources based on HMIS indicator results; and use of information as criteria for annual performance appraisals.
There is need for comprehensive capacity building interventions at the individual, organizational as well as system level. Critical focus areas in capacity building are data management and data quality assurance systems. Technical partners are providing support for capacity development through an on-line curriculum for routine health information set-up by MEASURE Evaluation in 2017.
Contents
Source chapter
The complete chapter on which we based this page:
Lippeveld T., Azim T., Boone D., Dwivedi V., Edwards M., AbouZahr C. (2019) Health Management Information Systems: Backbone of the Health System. In: Macfarlane S., AbouZahr C. (eds) The Palgrave Handbook of Global Health Data Methods for Policy and Practice. Palgrave Macmillan, London.
Additional resources
World Health Organization. Health facility and community data tool kit.
Aqil, A., Lippeveld, T., and Hozumi, D. PRISM Framework: A paradigm shift for designing, Strengthening and evaluating routine health information systems.
Belay H, Lippeveld T. Inventory of PRISM framework and tools: application of PRISM tools and interventions for strengthening routine health information system performance.
Lippeveld T. Routine health facility and community information systems: Creating an information use culture.
The Routine Health Information Network (RHINO) promotes behavioral change for better use of information in decision making.