Image by Tumisu from Pixabay
Why climate data for health?
Climate varies by location according to defined processes and has distinct cycles – diurnal, seasonal – as well as multi-year cycles such as those driven by El Niño.
What makes climate unique is that it is routinely measured – using land and sea observations, satellite-based remote sensing and global modelling outputs – modeled and predicted using standardized methods.This highly structured mass of data, in national and global repositories, provides hourly, daily, weekly or monthly information for most regions of the planet.
Health practitioners can use climate data to improve routine planning and surveillance, and preparedness activities to prevent and control specific diseases. They use the data to:
- Understand how climates impact health outcomes, for example, researchers found humidity wind and dustiness in the Sahel to correlate with the development of bacterial meningitis epidemics and proposed that information on specific climate conditions could trigger an early response.
- Identify populations at risk of climate-sensitive diseases. Health practitioners improve prevention, control and elimination programmes by mapping the geographic range of vectors and pathogens using environmental and climate covariates.
- Monitor and predict short-term and seasonal or year-to-year variations in incidence (including early warning systems for epidemics. In France, the devastating heat wave of 2003 led to the development of heat early warning systems that alert health workers and the general public to dangerous weather conditions.
- Monitor and predict longer term trends by assessing climate change impact and vulnerability. Researchers have used national climate data to assess the impact of warming temperature trends on the numbers of individuals at risk of malaria in the Ethiopian highlands.
- Assess the impact of climate sensitive interventions. Control programmes for diseases that are sensitive to rainfall or temperature need to account of climate when assessing the impact of their interventions. For example, a decline in malaria in East Africa preceded large-scale implementation of anti-malaria interventions in some regions; major droughts (2000, 2003, 2005) may have caused this early decline.
Sources of weather and climate data
In most countries, the National Meteorological and Hydrological Service (NMHS) place weather stations in strategic locations. Stations can be simple rain gauges or highly sophisticated automatic weather and climate recording equipment.
- Synoptic observations are snapshot hourly observations of weather, including wind direction, temperature, humidity, cloud cover.
- Climate observations are daily summaries of weather such as minimum and maximum temperature.
Local station operators compile daily or monthly reports which they send to the NMHS head office electronically, by radio or on paper.
The NMHS cleans, curates and archives the data in a central repository and uses the data to develop and test climate products which it shares with partners or clients free or for a fee. The NMHS may automatically communicate some daily data to the WMO’s Global Telecommunication System which provides the Global Weather Watch.
Other organisations and individuals may collect weather and climate data, for example schools, agricultural research centres, and farmers. Some health facilities manage weather stations for their locality. Records from these volunteer stations vary in quality and consistency and may not be integrated into the NMHS historical archives.
Ground-based observations are the gold standard for weather and climate data.
Global climate monitoring products are available free of charge, from national and international agencies.
Agencies such the United States National Aeronautics and Space Agency (NASA) and the European Centre for Medium Range Weather (ECMWF) create these products to complement (and sometimes integrate) ground-based data. These global products comprise:
- Satellite estimates for rainfall and temperature derive mainly from measurements taken by sensors on-board geostationary and polar satellites and incorporate limited ground observations.
- Reanalysis estimates of rainfall and temperature are obtained by systematically combining limited climate observations with model forecasts using data assimilation schemes and climate models.
- Sea Surface Temperatures (SST) indices. SST variations in the Atlantic, and the Indian and Pacific oceans influence climate on different time scales. El Niño-Southern Oscillation (ENSO) is the prominent mode of climate variability worldwide that operates on season to yearly time scales (two to seven years). Climate experts monitor SSTsin specific regions of the world’s oceans to indicate the current ENSO state (both El Niño and La Niña).
Choosing which data to collect
Researchers have to balance ease of access to climate data with data quality. The most accurate data come from local meteorological stations but, when they are not available, researchers resort to using global products (which may be calibrated using a subset of local observations).
Data errors for researchers to control for when analysing rainfall and temperature are:
- Rainfall data from near-by meteorological rain-gauge stations are usually aggregated over time, for example by week, month or season. Because rainfall is extremely variable in space and time – it can be raining heavily in one village while it is dry in a village five kilometres away – measurement accuracy depends on density and distribution of observing stations. Aggregation of rainfall data in time (for example, by month) means they are less variable geographically. Aggregated data, however, may contain errors that are invisible to the user, so it is essential to control for the quality of raw hourly or daily data; there are standard methods for doing this. Otherwise, to assess data quality, the user needs to know how the meteorological office cleaned the data, for example, how they handled missing daily observations and extreme values.
- Temperature varies by elevation, geography, latitude, proximity to water bodies, type of land cover, and so on. A common error in time-series of temperature data from weather stations is observable jumps in the records, frequently resulting from relocation of a station.Spurious break-points can influence analysis of temporal trends in temperature time series and their removal requires adjustments to the original data set. Ideally, the meteorological office would have recorded dates of any changes in station location and instrumentation to facilitate comparison with dates of any break-points.
Different gridded satellite rainfall estimates exist and may give different results for the same area. Which data set should be used? The best product will likely be the one that is calibrated using the most ground observations in the area of interest. Users can usually find this information in the documentation for the specific product.
Managing climate and health data
Data management and analysis tools range from simple Excel spreadsheets to sophisticated software requiring technical and statistical expertise. Commonly-used open source software include:
- The District Health Information System 2 (DHIS2) integrates climate data into its DHIS2 for health planning.
- Epi-InfoTM is a simple, freely available tool for epidemiological analysis which can incorporate climate data
- R-software is a relatively new and freely available programing language and software environment for statistical computing and graphics which researchers use increasingly for epidemiological studies including studies that integrate climate information into early warning systems.
- The International Research Institute Data Library at Columbia University in New York in which users can access, manage, analyse, and visualize large climate and environmental data sets and build tools designed to inform health decision-making.
Why model climate and health data?
Analysts model climate and health data using:
- Empirical statistical models to analyse the spatial and temporal distribution of disease in relation to climatic and environmental drivers – including identifying outbreaks and epidemics. Researchers cannot conclude a causal relationship unless they identify a mechanism to explain it.
- Mathematical mechanistic models, built on known or supposed relationship of external drivers to intrinsic processes in disease transmission, can explore the impact of the extrinsic climate drivers with other changes in the system. The causal relationship is assumed.
- Machine learning, for example, neural networks and other expert methods for forecasting the evolution of an ongoing epidemic. The models are thoroughly empirical in the sense that no attempt is made either to understand the causal nature of the relationships observed or, typically to take explicit account of the scientific context.
Presenting and interpreting data
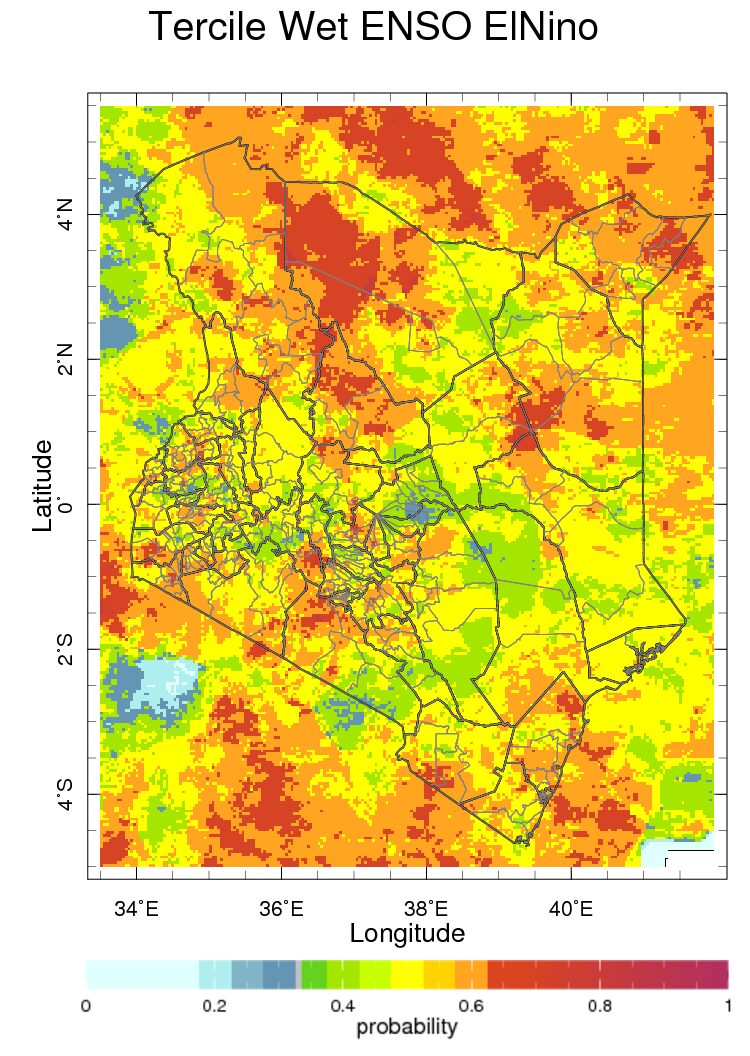
Map of the historical probability of seasonal monthly averages conditioned on El Niño in Kenya for seasonal average rainfall for October to December season.
Maps best visualise geographic distributions, seasonal patterns and year-to year-variations in risk over a region. But attractive as they appear, maps simplify reality.
Maps may describe the modelled relationship between climate predictors and health outcomes but often do not present the amount of uncertainty in the relationship. The model is unlikely to be predictive unless the relationship is plausibly causal, that is there is a known physical mechanism by which the predictor may influence the health outcome.
Choice of spatial and temporal scales influences interpretation. There are many ways to develop epidemic thresholds which give very different results. Although there are usually optimal spatial or temporal resolutions that provide greatest specificity and sensitivity, researchers take into account data availability when choosing between models, and there may be political considerations.
It is important that researchers explain what the maps mean to health planners.
Three applications
Malaria
WHO has prepared a framework for malaria early warning systems (MEWS) incorporating seasonal forecasts, rainfall and environmental monitoring and health facility surveillance which forms the basis for development of MEWS in different regions of the world.
The following climate variables that can predict malaria outcomes weeks or months into the future.
- Heavy rainfall creates puddles where malaria transmitting mosquitoes lay eggs, and increases humidity which promotes their survival. Satellite estimates and local station data predict epidemics weeks to months ahead.
- Rising temperature, especially in temperate or highland areas, hastens development of juvenile mosquitoes and reduces the time for parasites to develop in their female mosquito host, increasing the potential for epidemics.
- High humidity reduces evaporation of breeding sites, so they stay productive for longer and increases the likelihood that adult mosquitoes will survive long enough to transmit disease. Meteorological stations provide the only quality near real-time humidity measurements for early warning. It is difficult to interpolate humidity accurately between weather stations.
- Sea surface temperatures predict climate anomalies in certain regions and seasons where there are physical mechanisms that connect SSTs with local rainfall – for example East African short rains. SSTs are available in near real-time and readily accessible as global products.
Thomson et al. provide a detailed description of available climate products for use in malaria control and elimination.
Zika virus (ZIKV)
Zika virus (ZIKV) epidemics emerged in Latin America and the Caribbean in 2015 during a period of severe drought and unusually high temperatures which, Muñoz et al. showed to result from a combination of natural climate variability and long-term changes.
High rainfall provides more outdoor breeding sites for ZIKV mosquito vectors (predominantly the container breeder Aedes aegypti) and drought years may lead to greater water storage and more domestic breeding sites.
Warming temperatures increase development rates of both vector and virus. The study concluded that climate is a significant driver of seasonality, year-to-year variability and longer-term trends in the geographic distribution of ZIKV and other arboviruses transmitted by Aedes mosquitoes. The authors recommended that public health officials work with climate experts to use climate information to improve the timing and targeting of ZIKV interventions.
Climate, flooding and malnutrition
The 2015 Global Nutrition Report highlighted the risk of climate variability and changes in nutrition among vulnerable populations.(20) In Bangladesh, extreme flooding, driven in part by changes in global sea surface temperatures (SSTs) affects rice production, rice prices, and child malnutrition.
A preliminary study undertaken for the report indicated that seasonalrice production increases with the extent of the annual flood to a point after which flooding damages the crop. Monthly percentages of underweight children showed positive association with high prices and flood extent.
These results are consistent with evidence that high rice prices following production shocks (including those associated with climate events) strongly associate with people’s choices to spend less on non-rice foods (with higher densities of micronutrients) and increases in the number of underweight children.
A checklist
Questions for a planner who wants to coordinate climate data systems with health information data on a routine basis.
- Which problem/s do you want to address with climate data?Is it the identification of districts and villages that you need to target for indoor residual spraying? Or is it to better to estimate the number of patients with specific needs (for example, drugs, vaccines) that a district health facility might expect in the coming weeks?
- Who will use the data? Who are the stakeholders? Will you make your predictions publically available? How will you present the information to stakeholders? Developing the technical capacity for early warning must to go hand-in-hand with developing institutional capacity to use the information.
- Where do you want to collect and use the data? Do you want to set up systems in specific localities, a large region or the entire country? It is often useful to undertake a pilot in a small area but climate impacts may be easier to observe over a larger area with aggregate data.
- What climate data do you need and where will you find the data? Will you access data from local meteorological stations or use global products? If the data are locally acquired, are they open access? Can you set-up a data sharing policy? Is there a cost attached? Are suppliers of climate information able to provide data consistently and promptly?
- How will you manage the climate informed system? Consider approaches to integrating climate data with health data, for example the types of software needed and their compatibility with systems in current use. Do you need to appoint experts or to train staff to understand and use the information operationally?
Challenges and innovation
While many in the climate and health communities recognise the benefits of incorporating climate data and information into health decision-making, they face challenges in making this happen. The two communities have long-established methods for managing data within their sectors but have less experience in sharing data with each other.
A significant challenge is lack of access to quality data from country meteorological agencies. As a consequence, local and international users turn to freely available global products despite their quality limitations. Global products contain a fraction of the detail of locally recorded NMHS observations, and users may not fully understand their relevance and quality.
A remaining challenge is the capacity of health practitioners to value, collect and analyse climate data in health. Ministries of health need to partner not only with climate experts but with academic epidemiologists with technical skills. Few health training initiatives, whether in public health, medical or nursing schools, include a practical understanding of climate in their curricula. New initiatives are emerging that bring awareness of climate change, and knowledge about climate data and information to the health sector. The Global Consortium on Climate and Health Education, launched in February 2017, focuses on integrating climate into core curricula of today’s health professionals.
Contents
Source chapter
The complete chapter on which we based this page:
Thomson M., Lyon B., Ceccato P. (2019) Climate Matters in Health Decision-Making. In: Macfarlane S., AbouZahr C. (eds) The Palgrave Handbook of Global Health Data Methods for Policy and Practice. Palgrave Macmillan, London.
Additional resources
The Enhancing National Climate Services (ENACTS). ENACTS works directly with the NMHS to combine observations from the national observation network with global products – that is, satellite estimates for rainfall, and digital elevation models and reanalysis products for temperature. ENACTS also develops data and derived products and disseminates them via maprooms on the websites of the NMHSs.
Climate Information for Public Health. This is an open access book edited by Madeleine Thomson and Simon Mason.